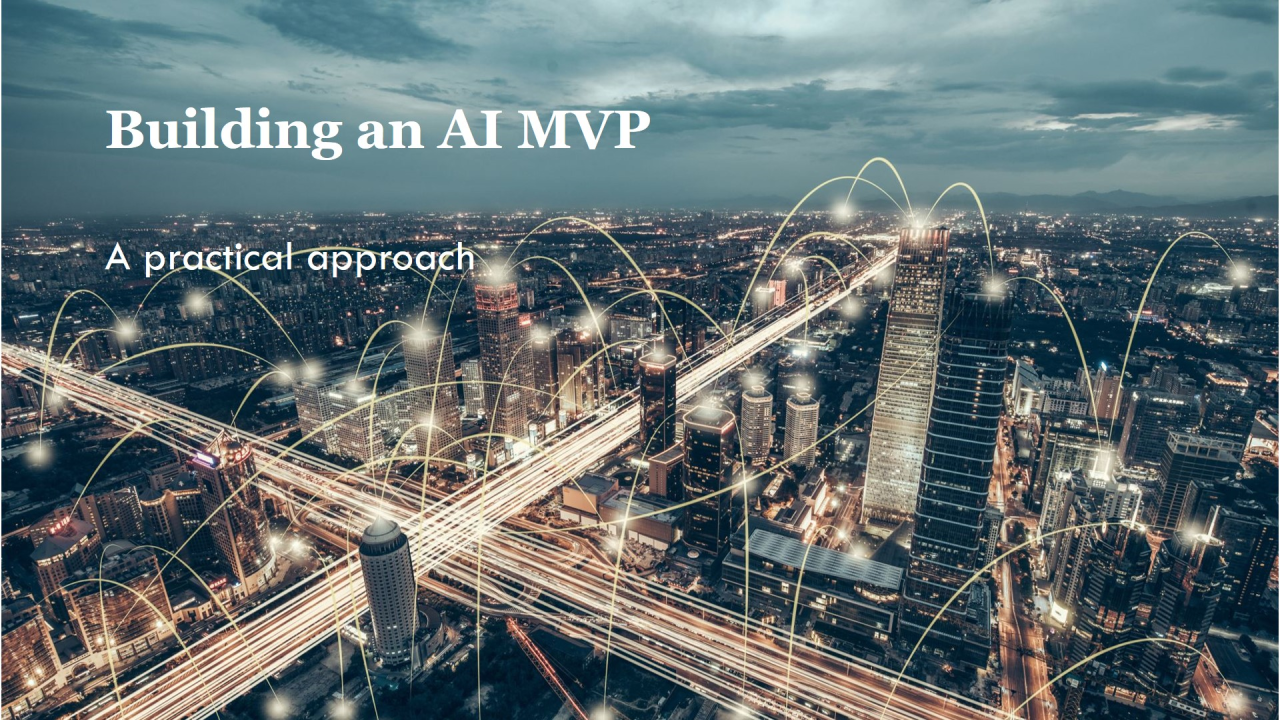
Building an AI MVP: A Practical Approach
As artificial intelligence (AI) continues to transform industries, many companies are looking to incorporate AI capabilities into their products and services. However, the process of building an AI-powered minimum viable product (MVP) can seem daunting. Here are some practical steps to help you get started:
- Define clear objectives and use cases: Identify specific business problems that AI can solve, focusing on high-impact areas where AI can provide tangible benefits.
- Choose appropriate AI technologies: Select the right AI approaches (machine learning, natural language processing, computer vision) based on your specific use cases and available data.
- Focus on data strategy: Assess what data you have and what you need. Create a plan for data collection, cleaning, and preparation as the foundation of your AI solution.
- Start small and iterate: Build a focused MVP that solves a specific problem well, rather than attempting complex features that delay your market entry.
Key Components of an AI MVP
- Data pipeline: Establish reliable data collection, storage, and preprocessing systems that can grow as your solution matures.
- AI model selection: Choose between building custom models, using pre-trained models, or leveraging AI services depending on your specific requirements.
- Evaluation metrics: Define clear success metrics for your AI components to measure performance and guide improvements.
- Feedback mechanisms: Implement ways to collect user feedback and model performance data to drive continuous improvement.
The XurLabs Approache
At XurLabs, we have developed a streamlined approach to building AI MVPs that balance innovation with practical delivery.
Here's how we approach AI product development:
- Technical feasibility assessment: We evaluate whether the proposed AI solution is technically feasible with current technology and available data.
- Rapid prototyping: We create proof-of-concept models to validate core AI functionality before committing to full development.
- Scalable architecture: We design solutions that can scale from MVP to enterprise-grade systems as adoption and requirements grow.
- Responsible AI practices: We incorporate ethical considerations, bias testing, and transparency measures from the beginning.
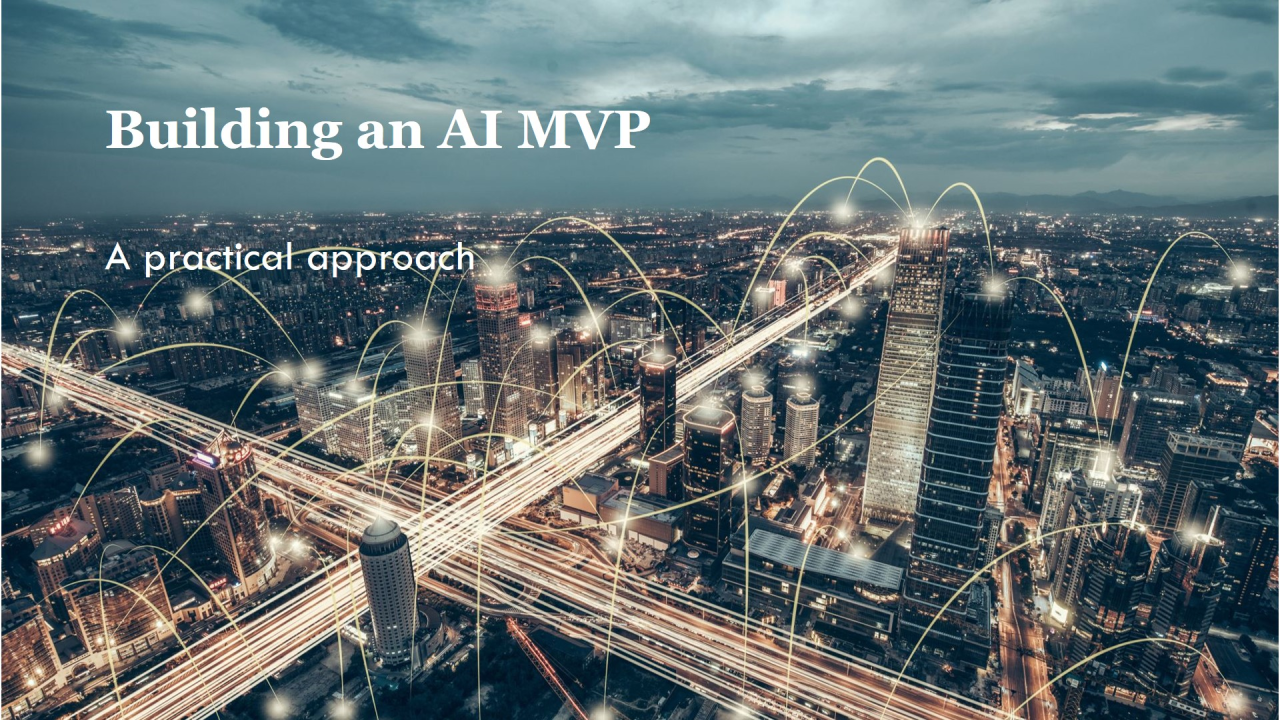
Conclusion
Building an AI MVP doesn't have to be overwhelming. By focusing on clear use cases, starting with core functionality, and implementing a systematic approach to development, you can bring AI-powered products to market efficiently. The key is balancing technical innovation with practical business value.
Ready to transform your business with a well-designed AI MVP?
Contact us today to learn more about how we can help you achieve your AI product goals.